In addition to retrieving internal knowledge from BigQuery, Cloud Storage, and Vector Search, we can improve our RAG system by integrating Google Search API to fetch the latest web-based information.
Step 1: Enable Google Custom Search API
1️⃣ Go to the Google Cloud Console → Google Custom Search API
2️⃣ Click Enable API
3️⃣ Generate an API Key:
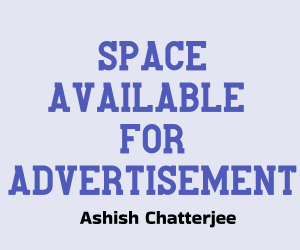
- Go to Credentials → Create API Key
- Copy the generated key for later use
4️⃣ Create a Custom Search Engine: - Go to Google Programmable Search Engine
- Click New Search Engine
- Add relevant websites or use
*.google.com
for general search - Copy the Search Engine ID
Step 2: Integrate Google Search API in RAG System
2.1 Install Required Libraries
Run the following command in your environment:
bashCopyEditpip install requests google-search-results
2.2 Fetch Latest Information from Google Search
Modify the rag-query
function to fetch top-ranked web articles based on the query.
pythonCopyEditimport requests
from flask import Flask, request, jsonify
# Google Search API credentials
GOOGLE_API_KEY = "your-google-api-key"
SEARCH_ENGINE_ID = "your-search-engine-id"
def google_search(query):
""" Fetch top search results using Google Custom Search API """
url = f"https://www.googleapis.com/customsearch/v1?q={query}&key={GOOGLE_API_KEY}&cx={SEARCH_ENGINE_ID}"
response = requests.get(url)
search_results = response.json()
# Extract snippets from top 3 results
snippets = [item["snippet"] for item in search_results.get("items", [])[:3]]
return " ".join(snippets)
# Flask API
app = Flask(__name__)
@app.route('/rag-query', methods=['POST'])
def rag_query():
data = request.json
query = data["query"]
# Retrieve internal knowledge (BigQuery + Vector Search)
query_embedding = model.encode([query]).tolist()
neighbors = index.find_neighbors(queries=query_embedding, num_neighbors=2)
retrieved_docs = [fetch_document(n.id) for n in neighbors]
# Retrieve external knowledge (Google Search API)
web_results = google_search(query)
# Combine all sources
context = " ".join(retrieved_docs) + " " + web_results
prompt = f"Use the following context to answer the query:\n{context}\n\nQuery: {query}"
# Generate response using LLM
response = llm.predict(prompt)
return jsonify({"response": response.text})
if __name__ == '__main__':
app.run(port=8080)
Step 3: Deploy the Enhanced RAG System
Once modified, deploy the enhanced API to Google Cloud Functions:
bashCopyEditgcloud functions deploy rag-query \
--runtime python310 \
--trigger-http \
--allow-unauthenticated
Now, when a user asks:
“What are the latest AI trends?”
The system will:
✅ Retrieve internal knowledge from BigQuery & Vector Search
✅ Fetch real-time information from Google Search
✅ Use an LLM (PaLM/Gemini) to generate a high-quality response
Use Cases of Google Search-Enhanced RAG
🔹 Finance & Market Research → Fetch latest stock updates & regulations
🔹 Cybersecurity Alerts → Get real-time security vulnerabilities
🔹 Healthcare & Medical Research → Provide up-to-date disease trends
🔹 Technology & AI News → Retrieve the latest advancements in ML/AI
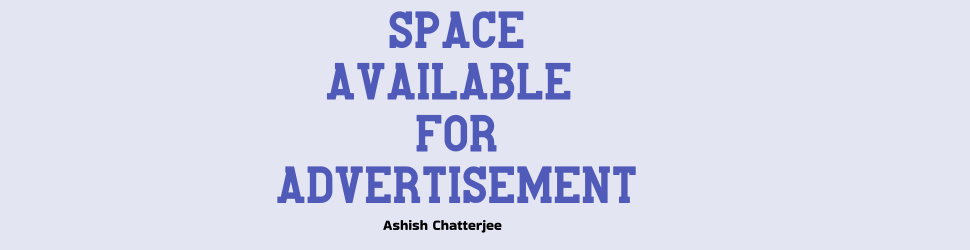
GIPHY App Key not set. Please check settings